Enhancing Bangla NER with Comprehensive Data and Advanced Models
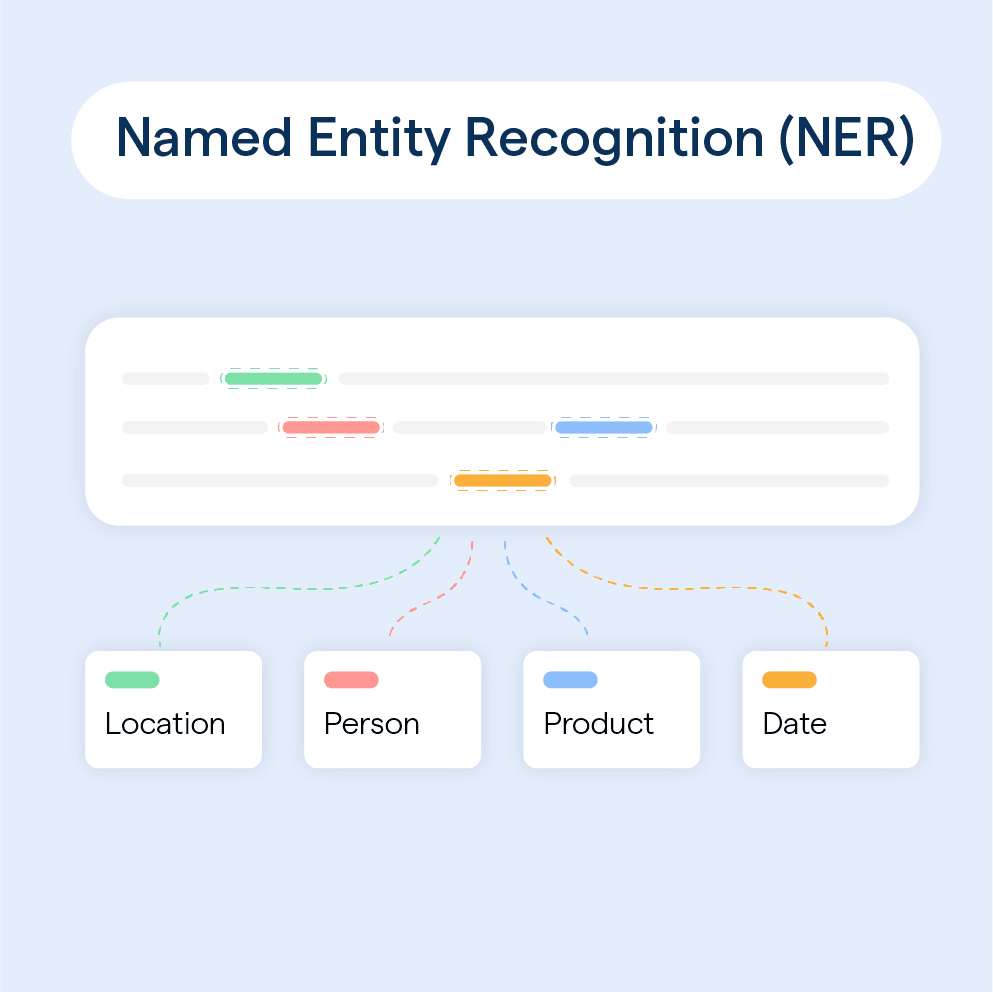
Named Entity Recognition (NER) is a crucial component of Natural Language Processing (NLP) for the Bangla language, facilitating the extraction of essential information for various applications such as information extraction, question answering, and sentiment analysis. Despite its importance, Bangla NER faces significant challenges due to the limited availability of labeled data and the unique complexities of the language. Bangla alphabets, characterized by complex ligatures, pose additional difficulties for models to accurately parse the text. For instance, some models often struggle to differentiate between similar-looking characters such as “ত” and “৩”, owing to the small representation of Bangla in their training data.
To address these challenges, our project investigates the use of models specifically designed for Indic languages, including Bangla. Unlike other multilingual models, these models have been trained extensively on large Indian text corpora, enabling them to handle the intricate ligatures and unique features of Bangla more effectively. By leveraging the inherent understanding of “মাত্রা”, “অর্ধমাত্রা”, and “পূর্ণমাত্রা” without additional training, these models demonstrate a superior capability in encoding Bangla text.
Our research involves fine-tuning these models with a Bangla dataset, revealing that they significantly outperform other multilingual models in NER tasks. This finding challenges the current reliance on general-purpose models in existing NER systems and highlights the potential benefits of adopting language-specific models for Bangla NER. Furthermore, we are addressing a notable gap in existing Bangla NER datasets by incorporating time and date data, thereby enhancing the dataset’s comprehensiveness and utility. Through this project, we aim to advance the accuracy and effectiveness of Bangla NER, paving the way for more robust and reliable NLP applications in the Bangla language.